Embedded AI
Josef Ressel Zentrum
This JR Center is researching new methods of retraining that dynamically adapt machine learning models (AI models) directly on distributed resource-limited devices (RLDs). This should enable them to make autonomous, self-learning decisions.
AI models and algorithms are only as good as the data they have been trained with: After all, they adapt their approach based on this “wealth of experience” and make decisions that seem sensible in view of the training data. However, such models are always highly specialized: If, for example, an AI-controlled industrial robot is tasked with attaching screws and “knows” exactly how screws should look and be used, it cannot simply “rethink” on its own if it suddenly has to work with nuts instead.
In such cases of constantly changing system and environmental parameters, one speaks of “concept drift” or “model drift” and would currently have to completely retrain the underlying model in order to adapt said robot to its new work objects, for example.
However, when it comes to a network of distributed resource-limited devices, such as a network of specialized robots, this is not only time-consuming and cost-intensive, but also a particularly complex undertaking. Typically, such re-training requires a constant exchange of all sensors with a powerful cloud system, which adapts or re-trains the model when the data changes and then redistributes the model to all participating devices. It becomes even more difficult if certain data cannot or must not be sent to the server in the cloud, for example for data protection reasons in the healthcare sector.
The team at the JR Center is therefore developing a methodical and efficient framework to distribute models in industrial applications to RLDs and to be able to adapt them at runtime instead of having to use powerful server systems with large memory and high computing capacities for complex re-training, as is currently the case.
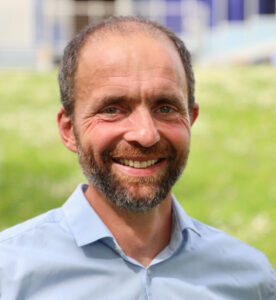
head of department:
Prof.(FH) DI Dr. Florian Eibensteiner
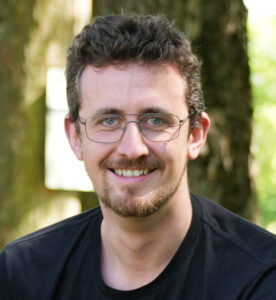
Deputy:
Phillip Petz, MSc.
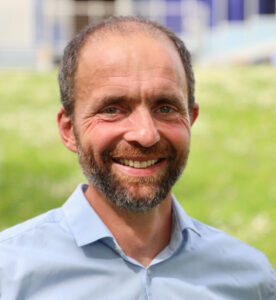
Leiter:
Prof.(FH) DI Dr. Florian Eibensteiner
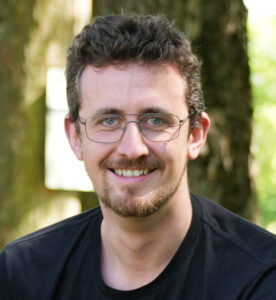
Stellvertreter:
Phillip Petz, MSc.
In addition, in collaboration with the CD Laboratory for Embedded Machine Learning (see links), an evaluation of the adaptation and optimization of various models with regard to their ability to run on RLDs is to be carried out.
The overall objective of the JR Center is therefore to retrain AI algorithms on embedded systems for changing environments and thus enormously multiply the possible applications of AI: This will enable the use of AI systems on distributed microprocessor and microcontroller systems with limited resources while maintaining privacy and real-time requirements.
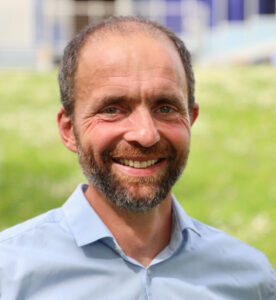
Leiter:
Prof.(FH) DI Dr. Florian Eibensteiner
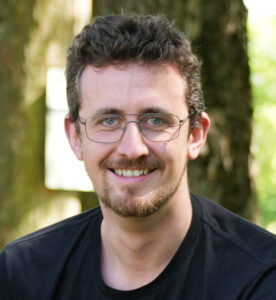
Stellvertreter:
Phillip Petz, MSc.
Kontakt
FH OÖ Forschungs und Entwicklungs GmbH
Embedded Systems Lab
Adresse:
Softwarepark 11
4232 Hagenberg
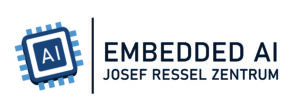
Contact
FH Upper Austria
Research and Development GmbH
Embedded Systems Lab
Adresse:
Softwarepark 11
4232 Hagenberg
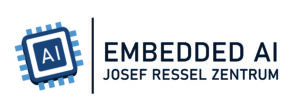
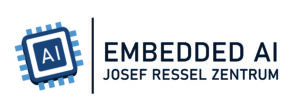